Towards assessing attentional states
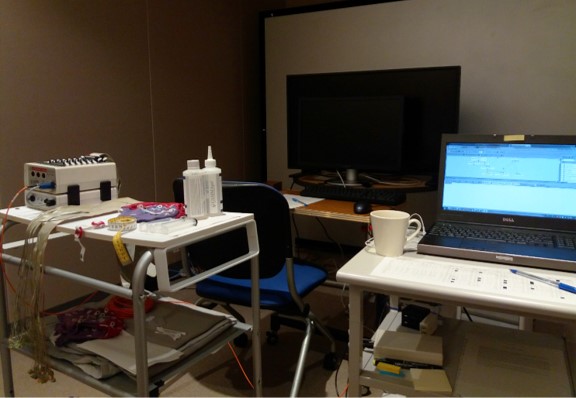
General context:
Mental-Imagery based Brain-Computer Interfaces (MI-BCIs), which are neurotechnologies enabling their users to command external digital devices, such as neuroprostheses or videogames, only by performing mental imagery tasks, such as imagining an object rotating in space [Clerc et al., 2016].
While MI-BCI users perform mental-imagery tasks, their brain activity is recorded (often using electroencephalography), processed and translated into commands for the system.
To control MI-BCI, the user first needs to train to produce a reliable brain activity recognizable by the system. During the training, a feedback, which is an information provided to a learner regarding aspects of the performance or understanding of the task/skills to learn, is provided by the MI-BCI system.
The feedback is a fundamental component of the MI-BCI training [Lotte et al., 2013].
However, the literature and experimental results suggest that current MI-BCI training protocols, including the feedback, are inappropriate to acquire MI-BCI related skill [Jeunet et al., 2016].
Some of the users’ traits and states, i.e., respectively their stable and temporary personal characteristics, are correlated with the ability to acquire MI-BCI skills.
Tensed and non-autonomous users, for example, tend to have lower control over MI-BCI than relaxed and autonomous users [Jeunet et al., 2016].
The current lack of reliability of MI-BCI limits the development of the technology outside of research laboratories.
Timing of feedback:
Little information exists in the MI-BCI literature regarding the timing that feedback should have, i.e., its moment and frequency of presentation.
However, the more frequent the feedback is, the more attentional resources are necessary to process it. Previous studies indicate that participants' attentional traits and states have an influence on their ability to control MI-BCI [Jeunet et al., 2015].
My hypothesis is that continuously adjusting the frequency of feedback according to the users’ attentional states would improve the training outcome.
“Attention” is a generic word which according to the model of van Zomeren and Brouwer (1994) encompasses alertness and sustained attentions, referring to the intensity of attention (i.e., strength), as well as selective and divided attentions, referring to its selectivity (i.e., amount of monitored information).
Before being able to test this hypothesis, the ability to distinguish the different types of attention from one another had to be assessed.
Assessing the EEG characteristics of attentional states:
In a study led in Japan near Tokyo during a 6 months internship at the RIKEN Brain Science Institut, I asked 18 participants to perform different tests each related to one of the four types of attention from the model of van Zomeren and Brower (see Picture of our setup).
During each test, participants had to react as fast as possible – by pressing the space bar of a keyboard – to the appearance of target stimuli, while we recorded their brain activity.
The tests and types of attention were differentiated by the modality of presentation of the stimuli, number of distractors, presence of a warning before the stimuli and the length of the task.
The results indicate that it is possible using only EEG data to distinguish with a promising accuracy the attention states described in the van Zomeren and Brower model and tend to validate the latter.
Such a result should be used in the future to adapt the timing of the feedback to the attentional state of the users.
All of the details regarding this experiment can currently be found in my PhD manuscript